(Ab)normal Language: HSE Researchers Present Digital Tools for Assessing Mental Health Problems
Often, individuals with neurological or mental disorders exhibit distinctive language patterns. In modern clinical practice, digital tools can play a significant role in supporting language therapy and rehabilitation for persons with language disorders. Additionally, in the future, digital tools could assist healthcare specialists in assessing the severity of symptoms associated with such disorders.
As part of the 'Human Brain Resilience: Neurocognitive Technologies for Adaptation, Learning, Development and Rehabilitation in a Changing Environment' Strategic Project, a joint research seminar was held, focusing on computational linguistics and the development of diagnostic and therapeutic methods for individuals with neurological and psychiatric disorders.
According to Mariya Khudyakova, Director of the Centre for Language and Brain Studies at the HSE Campus in Nizhny Novgorod, language corpora serve as essential tools for researching and developing such methods. These databases are collections of texts, speech samples, or video recordings in various languages, used by linguists to analyse linguistic phenomena. Language corpora can be categorised into general and specialised types.
For example, clinical language corpora include not only speech recordings but also demographic information about patients, their diagnoses, medical histories, and the results of various tests and assessments.
Today, several challenges exist in compiling clinical language corpora, including ethical considerations, secure storage of audio recordings and personal data—such as medical information—and a lack of sufficient data for machine learning applications.
Nevertheless, these databases offer unique opportunities for both quantitative and qualitative analysis, and for detecting patterns and trends. For example, it is possible to identify speech errors and abnormalities characteristic of patients with specific neurological or psychiatric disorders.
Many tests used to assess language and cognitive abilities include a verbal fluency task, in which the subject is asked to name as many items as possible within one minute, such as animals or words starting with the letter M. Based on the test results, researchers evaluate not only the number of words generated but also the semantic clusters and the patterns of pauses in the person's speech.
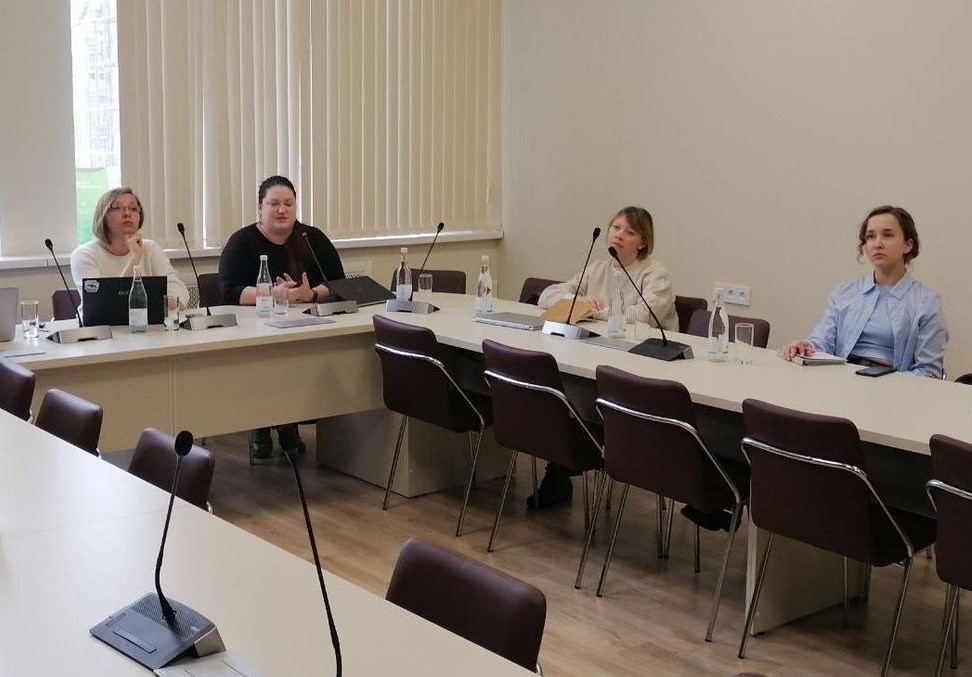
Scientists have already successfully identified several notable patterns. For example, individuals with depression recall fewer words during a verbal fluency test compared to healthy subjects. The 'flight of ideas' characteristic of the manic phase of bipolar disorder is reflected in a high frequency of switches between topics, while clustering is less pronounced in those with thought disorders.
Researchers at the HSE Campus in Nizhny Novgorod conducted a pilot study using automatic analysis of verbal fluency in patients with psychiatric disorders, a control group, and individuals with aphasia. Aphasia is a disorder characterised by impaired language production and comprehension. It arises from organic damage to the language-processing areas of the cerebral cortex, often resulting from injuries, tumours, strokes, or similar conditions.
The researchers analysed verbal fluency using 400 recorded samples and incorporating medical information, questionnaire responses, and mental health rating scales. Currently, there is insufficient data to implement the model in clinical practice, but plans for the future include developing a specialised mobile application and testing it in psychiatric clinics in Moscow and Nizhny Novgorod.
Anna Khomenko, Senior Research Fellow at the Centre for Language and Brain Studies of the HSE Campus in Nizhny Novgorod, discussed another study aimed at diagnosing mental disorders based on the analysis of participants' speech. According to her, many existing models consider only the acoustic characteristics of the audio signal. While this approach makes them relatively universal and language-independent, it also limits their ability to account for the full range of speech data.
This project is based on methods of attribution linguistics and speech profiling, which are used to create a linguistic profile of individuals with schizophrenia spectrum disorders and affective disorders.
'Using these methods, we aim to create a model capable of identifying speakers with symptoms of thought and mood disorders based on speech transcripts,' she says.
In the future, speech profiles could be used in an automated diagnostic model to identify individuals with mental disorders.
As part of the project, the researchers analysed the oral speech of 199 individuals diagnosed with schizophrenia, schizotypal disorder, schizoaffective disorder, borderline personality disorder, primary depressive episode, recurrent depressive disorder, and bipolar affective disorder. They identified statistical data for various parameters, including word and sentence lengths, dynamism and objectivity coefficients, readability, part-of-speech characteristics, vocabulary volume and richness, and more. They then analysed the clustering of vocabulary through speech material vectorisation and, using mathematical statistics, identified the parameters most significant to the language of each group of subjects.
The study reveals that the language of individuals with mental disorders generally tends to be more basic and less dynamic, consisting of shorter sentences and primarily concrete words. However, despite the lower levels of complexity, such texts demonstrate greater lexical diversity and higher coherence. While the language of the control group is more active and varied in terms of actions and sentence length, the language of individuals with mental disorders, though simpler, tends to be more predictable and concrete.
The specific language characteristics of each group have also been identified. Thus, patients with depressive disorders exhibit high text complexity, while individuals with schizophrenic disorders produce texts that are easier to comprehend. The language of patients with personality disorders and depressive episodes is characterised by high lexical diversity, reflecting a rich vocabulary. In contrast, individuals with bipolar affective disorder and borderline personality disorder exhibit lower lexical diversity. Persons with schizotypal and schizoaffective disorders demonstrate high concreteness, while patients in depressive states tend to use more abstract language.
The language of individuals with personality disorders tends to be more evaluative and active, while in patients with borderline personality disorder, it is less dynamic.
Patients with schizoaffective disorder and schizophrenia exhibit high coherence in the texts they produce.
Olga Lyashevskaya, Professor at the HSE School of Linguistics, shared her experience in developing applications for the rehabilitation and skills training of individuals with speech and language impairments, as well as vision and hearing loss. These applications can be used independently by patients and serve as a valuable supplement to sessions with specialists. The speaker gave three examples of applications developed at HSE University.
SLON, a mobile application for naming practice using pictures, is designed for the rehabilitation of patients recovering from a stroke (anomia, dysarthria) with mild to moderate impairments. Patients use the application independently and receive feedback and can also export data to a specialist.
An online application for teaching the 10-finger typing method is designed for elderly people with partial vision loss. The texts in this application have enhanced readability features (such as background and font adjustments), and the data is used to track progress in typing. The dyslexia correction mobile application helps address phonemic (phoneme discrimination by ear), optical (letter mixing, reading order), semantic (understanding and connections between words), and agrammatic (coordination) difficulties.
Lala Kasimova, Head of the Department of Psychiatry at PRMU, advised the researchers to focus on defining the mental norm and identifying its variations. 'There are many abnormalities that fall within the norm,' the psychiatrist explained.